Unlocking the Power of Video Annotation Tools for Machine Learning
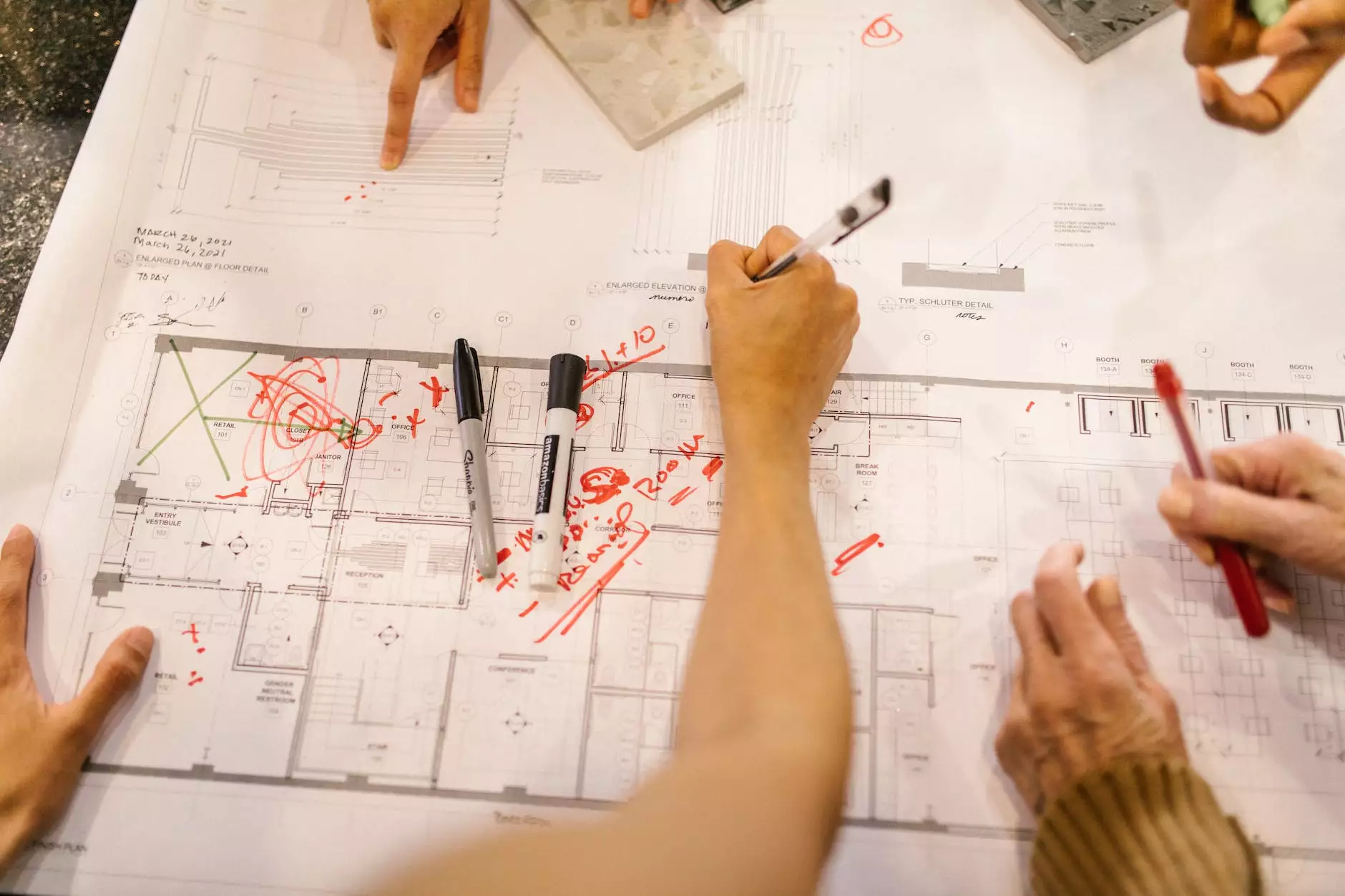
Video annotation tools for machine learning have become essential elements in the arsenal of data scientists and machine learning engineers. In an era where artificial intelligence (AI) is integral to advancements in multiple industries, the demand for high-quality training data has never been higher. This article explores the significance of video annotation tools, how they enhance machine learning applications, and what makes KeyLabs.ai a standout choice in the market.
Understanding Video Annotation
Video annotation refers to the process of labeling clips in a video format. It assists in providing instances of different objects, activities, or events within a video. This systematic process allows machine learning algorithms to understand and interpret visual information effectively. Without annotated video datasets, training machine learning models would be an intricate challenge.
The Importance of Video Annotation in Machine Learning
Video data is inherently complex, consisting not just of static images but also of dynamic sequences and interactions. The importance of video annotation for machine learning lies in its ability to:
- Facilitate Supervised Learning: Video annotation generates labeled datasets that are crucial for supervised learning, enabling algorithms to learn from examples.
- Improve Recognition Accuracy: Accurate annotations lead to precise recognition and prediction outcomes, making the models more reliable.
- Support Contextual Understanding: Annotations provide context, empowering AI to understand the nuances of actions, behaviors, and interactions within videos.
- Enhance Real-time Applications: In applications like autonomous driving and surveillance, precise video annotations can significantly impact performance in real-time decision-making.
Types of Video Annotations
Understanding the different types of video annotations is crucial for choosing the right tool for your machine learning needs. Below are the most common types:
- Object Detection: This involves identifying and labeling specific objects within a video frame.
- Action Recognition: Annotates actions or behaviors observed in video sequences.
- Semantic Segmentation: Breaks down frames to label pixels, allowing models to understand boundaries and shapes.
- Keypoint Annotation: Focuses on identifying significant points on objects, helpful in gesture and pose recognition.
Advantages of Using a Video Annotation Tool
Leveraging the right video annotation tool for machine learning provides several advantages:
- Efficiency: Automated workflows can dramatically reduce the time and effort required for video annotation.
- Scalability: Tools are designed to handle various scales of data, from small projects to massive datasets required by enterprise applications.
- Collaboration: Many tools offer features for teams to collaborate in real-time, enhancing productivity and accuracy.
- Quality Control: Integrated review mechanisms ensure that annotations are accurate and meet project standards.
The Role of KeyLabs.ai in Video Annotation
KeyLabs.ai has distinguished itself as a leader in the field of data annotation. Specifically, their platform offers robust features tailored for effective video annotation:
1. User-Friendly Interface
KeyLabs.ai provides a user-friendly interface that simplifies the entire annotation process. Users can easily navigate the platform, making it accessible for both seasoned professionals and beginners alike.
2. Advanced Annotation Techniques
The platform supports various sophisticated annotation techniques, including:
- Automated Tracking: Automatically identifies and tracks objects across video frames.
- Multi-Label Support: Annotate multiple object types within a single video frame, catering to complex scenarios.
- Real-Time Collaboration: Enables teams to work together seamlessly, with changes instantly visible to all users.
3. Integration with Machine Learning Workflows
KeyLabs.ai’s video annotation tool can integrate smoothly with existing machine learning workflows, ensuring that annotated data can be directly utilized. This feature significantly accelerates the model training process and optimizes performance.
Best Practices for Video Annotation
To achieve the best results from your video annotation efforts, consider the following best practices:
- Define Clear Objectives: Have a clear understanding of what you need the annotations for, whether it’s for action recognition or object detection.
- Consistent Labeling Guidelines: Create and maintain a consistent set of annotation guidelines to ensure uniformity across the dataset.
- Regular Quality Checks: Implement routine quality control measures to identify and correct errors in annotations.
- Train Your Crews: Ensure that your annotation team is well-trained on the specific requirements of the task.
The Future of Video Annotation and Machine Learning
The future of video annotation is incredibly promising, particularly as advancements in AI continue to evolve. As the complexity of machine learning models increases, so too does the need for high-quality, precise annotated data. Here are a few trends to watch:
- Automated Annotation: The rise of machine learning algorithms capable of performing initial annotation on videos, speeding up the process.
- Enhanced AI Models: As AI improves, models will become capable of learning from fewer annotated samples, reducing the annotation burden.
- Cross-Domain Applications: Video annotation will find applications in sectors beyond traditional AI, including augmented reality, virtual reality, and robotics.
Conclusion
In conclusion, video annotation tools for machine learning are not just a trend; they are a necessity for developing effective AI models. The process of annotating videos is crucial for teaching machines to understand and interpret complex visual data. With platforms like KeyLabs.ai, organizations can harness the power of video annotations, streamlining their machine learning projects and enhancing accuracy. As we move forward, the evolution of video annotation technology will undoubtedly shape the future of machine learning and AI.