Mastering Image Labeling: Enhancing Your Data Annotation Strategy
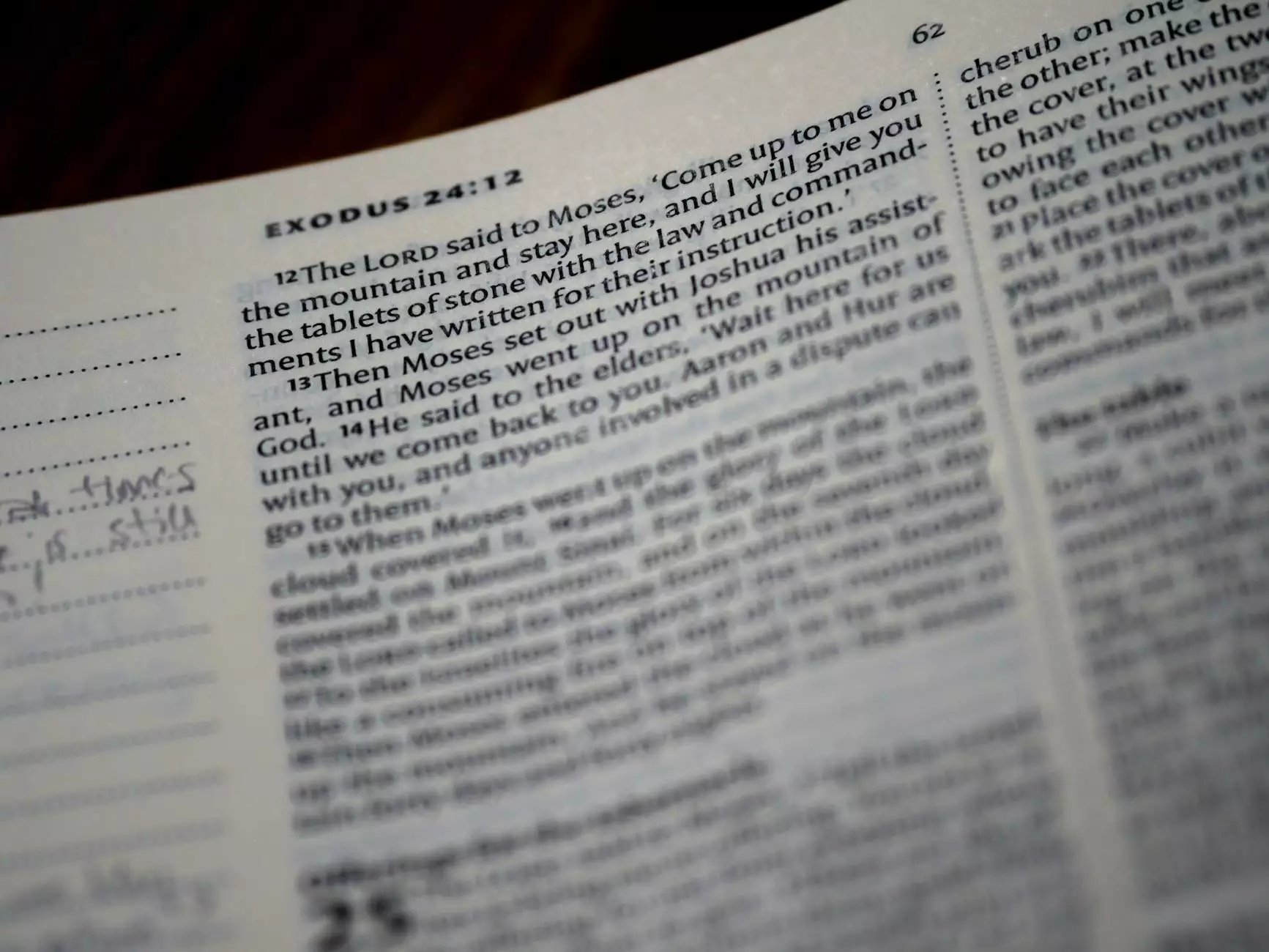
Image labeling has become a critical component in the realm of artificial intelligence and machine learning. With the increasing reliance on computer vision technologies, understanding the nuances of image labeling can significantly enhance the performance of your models. In this comprehensive guide, we will delve into the world of image labeling, explore its importance, and discuss how effective data annotation tools and platforms, such as those offered by Keylabs.ai, can streamline your processes.
Understanding Image Labeling
Image labeling refers to the process of identifying and annotating images to assist machine learning algorithms in recognizing and interpreting visual data. This practice is fundamental for various applications, including but not limited to:
- Image Classification: Assigning a category to an image.
- Object Detection: Identifying objects within an image and marking their locations.
- Semantic Segmentation: Classifying each pixel of an image into a category.
- Facial Recognition: Recognizing and verifying individuals within images.
The Importance of Image Labeling in Data Annotation
Image labeling is crucial for a myriad of reasons:
- Data Quality: High-quality labeled data is essential for training accurate models. Poorly labeled data can lead to model degradation.
- Model Performance: Accurate labeling directly influences the performance of AI models, ensuring high precision and recall rates.
- Scalability: Automated and semi-automated image labeling tools allow businesses to scale their annotation processes effectively.
- Cost Efficiency: By utilizing advanced tools like Keylabs.ai, companies can reduce the time and cost associated with manual annotation.
Choosing the Right Data Annotation Tool
When it comes to image labeling, the choice of data annotation tool is paramount. Here are some features you should consider when selecting a tool:
1. User-Friendly Interface
The tool should have an intuitive user interface that allows for easy navigation and usage, even for individuals who are not tech-savvy.
2. Customization Options
Your data annotation tool should allow you to customize label sets according to your specific needs, maximizing the relevance of the annotations.
3. Collaboration Features
As your team grows, a platform that enables seamless collaboration is vital. Look for tools that support multiple users and easy sharing of annotated datasets.
4. Automation Capabilities
Tools with machine learning integration can automate the image labeling process, reducing manual input and potential errors.
5. Support and Training
Choosing a provider that offers comprehensive support and training ensures that your team can make the most of the tool's capabilities.
Image Labeling Techniques: A Closer Look
There are various techniques for image labeling, each suited for different types of tasks:
1. Manual Labeling
This traditional method involves human annotators labeling images by hand. While it ensures high accuracy, it can be time-consuming and costly.
2. Automated Labeling
Machine learning algorithms can automate the labeling process. While this method is faster and more scalable, it may require initial training with well-labeled datasets.
3. Semi-Automatic Labeling
This hybrid approach uses algorithms to suggest labels which human annotators can then verify and correct, balancing efficiency and accuracy.
Best Practices in Image Labeling
Implementing best practices in image labeling can significantly impact your project's success:
1. Clear Guidelines
Provide your annotators with clear, concise guidelines on how to label images to ensure consistency across the dataset.
2. Regular Quality Checks
Conduct regular quality assurance checks to maintain high labeling standards and address any discrepancies promptly.
3. Use of Pre-trained Models
Leverage pre-trained models to bootstrap your annotation process. This can reduce the workload significantly in projects with large datasets.
4. Continuous Learning and Feedback
Foster an environment of continuous learning, where annotators can share feedback on the labeling process and improve over time.
Case Studies: Successful Implementations of Image Labeling
To illustrate the impact of effective image labeling, let's explore a few case studies:
1. Autonomous Vehicles
In the development of autonomous vehicles, accurate image labeling is crucial for object detection systems. Companies like Tesla have invested heavily in image labeling to train their AI systems to recognize pedestrians, road signs, and other vehicles, enabling safer navigation.
2. Healthcare Sector
Medical imaging has benefited immensely from precise image labeling. Hospitals and medical research facilities utilize image labeling to train AI models that can detect anomalies in X-rays or MRI scans, leading to earlier detection of diseases.
3. Retail Industry
Retail giants have adopted image labeling for visual search technologies. By labeling product images, they enable customers to search for items using pictures rather than keywords, enhancing user experience and engagement.
Future Trends in Image Labeling
The field of image labeling is continually evolving. Here are some emerging trends to keep an eye on:
1. Enhanced AI Integration
AI technologies are becoming increasingly integrated into data annotation platforms, enabling smarter automation and improved accuracy.
2. Real-Time Annotation
With advancements in technology, real-time image labeling is becoming a reality, allowing for instantaneous annotations that can be used immediately for model training.
3. Cloud-Based Solutions
Cloud-based data annotation tools are growing in popularity, offering flexibility and scalability while reducing the need for extensive on-premise infrastructure.
4. Community Annotation
Leveraging the crowd for image labeling through platforms that enable community engagement can lead to diverse insights and richer datasets.
Conclusion
In conclusion, mastering image labeling is essential for anyone looking to harness the power of machine learning and AI. With the right tools, practices, and strategies in place, businesses can ensure accurate, high-quality image annotations that will drive their projects to success. Platforms like Keylabs.ai provide comprehensive solutions to streamline the data annotation process, making it easier than ever to embark on your data-driven journey.
This guide serves as a cornerstone for understanding image labeling's significance and best practices in data annotation. Implement these insights today to elevate your business operations and stay ahead of the competition.